As the world continues to shift towards renewable energy, wind turbines have become a crucial part of the energy landscape. However, like any other mechanical system, wind turbines require regular maintenance to ensure optimal performance. This is where predictive maintenance comes into play, and where FAT FINGER, a digital workflow procedure builder, can make a significant impact. FAT FINGER empowers front-line teams to do their work correctly every time, by letting you build checklists, workflows, and digital procedures that unlock operational excellence.
With features like Drag & Drop Workflow Builder, Mobile & Desktop Workflows, Dashboards, Integrations, Augmented Reality, Connect IoT Devices, and Artificial Intelligence Coaching, FAT FINGER can help you build predictive maintenance digital workflows to ensure problems are gone even before they start. Request a demo today to see how FAT FINGER can revolutionize your wind turbine maintenance.
The Importance of Predictive Maintenance
Predictive maintenance is an engineer’s best friend and a powerful tool for optimizing platforms in a manufacturing facility. Instead of having scheduled maintenance done on a predetermined timeframe, predictive maintenance uses data and technology to specify when maintenance needs to be completed to prevent costly breakdowns accurately. It works by collecting data from a facility’s various components and then using it to determine when parts need maintenance, as well as use algorithms or Artificial Intelligence (AI), if necessary. Predictive maintenance can help save time and money, improve efficiency in the facility, and help ensure that safety standards are adhered to throughout the lifecycle of all machines.
Improving Wind Turbine Performance with Predictive Maintenance
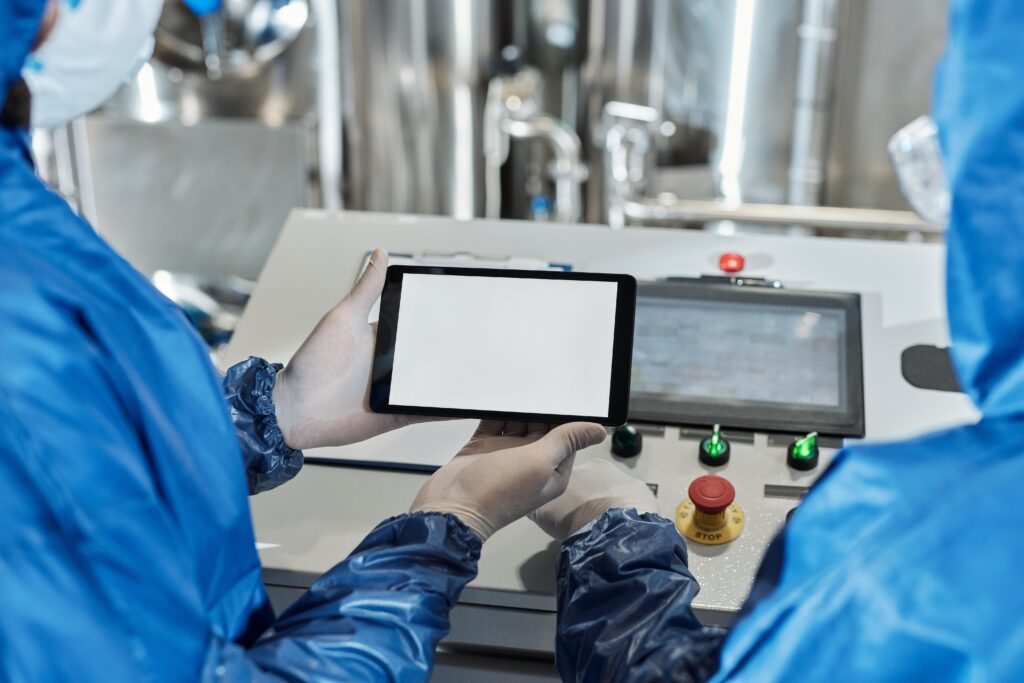
Wind turbines are complex machines with many moving parts, making them susceptible to wear and tear. Regular maintenance is essential to ensure they continue to operate efficiently and safely. However, traditional maintenance methods can be time-consuming and costly, often involving scheduled downtime that can impact energy production.
Predictive maintenance, on the other hand, uses data collected from sensors installed on the turbines to monitor their condition in real-time. This data can be analyzed to identify patterns and trends that may indicate a potential failure before it happens. This allows for maintenance to be scheduled at the most opportune time, minimizing downtime and maximizing energy production.
Case Study: Predictive Maintenance in Action
A great example of predictive maintenance in action is a wind farm in Texas, which used FAT FINGER’s predictive maintenance workflows to monitor their turbines. By analyzing data from the turbines, the team was able to identify a potential gearbox failure before it happened. This allowed them to schedule maintenance at a time when wind conditions were unfavorable for energy production, minimizing downtime and saving the company thousands of dollars in potential repair costs.
Conclusion
As the demand for renewable energy continues to grow, so does the need for efficient and effective maintenance strategies for wind turbines. Predictive maintenance, powered by digital workflow builders like FAT FINGER, offers a solution that not only improves the performance of wind turbines but also reduces costs and downtime. By leveraging data and technology, predictive maintenance allows for timely and accurate maintenance, ensuring that wind turbines continue to operate at their best, contributing to a greener and more sustainable future.
Ready to revolutionize your wind turbine maintenance? Create a predictive maintenance workflow for free on FAT FINGER or schedule a demo today.
Discover how to improve your wind turbine performance through predictive maintenance. Don’t wait for a breakdown to happen, anticipate it and prevent it. Learn more about our innovative solutions and how they can benefit your business. Schedule a Demo today and take the first step towards optimized wind turbine performance.